Machine Learning for Copycat Investors – Part 2
The first part of this series defines machine learning based alpha cloning strategies and the ways different market participants implement them, from hedge funds to ETFs and mutual funds. Part 1 reviews the constraints of scouring forms 4 and 13F regulatory filings to follow investor movements and explains why industry professional Warren Buffet advises against stock picking for retail investors leaving the development of ETF clone creation in the hands of financial institutions. Some of which still rely on outdated information from regulatory filings and news reports.
With the development of new AI-based methods, they no longer need to. Now, new methods can provide end-of-day or real-time information on long and short positions of institutional investors, giving clones a more complete view of the entire market. Institutional investors can use Fund Asset Inference to reveal the constituents of a specific portfolio and sentiment analysis to bolster existing knowledge and predict up or down trends in particular companies or entire industries.
This means ETFs run by institutional investors can implement cloning strategies that adjust in real-time instead of waiting on outdated information and can reveal short positions as opposed to relying on knowing only half of hedge fund movements.
Getting Around SEC Time Constraints
Sentiment Analysis
Sentiment analysis often uses natural language processing (NLP), a form of machine learning (ML) that can scan tweets, headlines, and other forms of media en masse for consensus views on investment activity. Although not robust enough as a standalone strategy, it is helpful when layered among other data mining strategies.
Since it is impossible for a human being to analyze all available text data in the media, different methods exist that are capable of scoring sentiment in seconds. Bag of Words (BoW) is a type of rating system that counts positive and negative words within in an article. Positive words are assigned the score of 1, while negative words are assigned –1 point. There are some drawbacks to this as the analysis ignores word order making sentence level analysis a more attractive option
S&P Global’s Market Intelligence Quantimental Research team using NLP has revealed that analyzing earnings calls for certain speech patterns can indicate an incoming decline in share prices. Good news is usually delivered with clarity, where bad news is veiled in discursive, round-about language. One of the companies that S&P predicted would go into decline after analyzing its earning call was Seagate Technology, which subsequently dropped 35% in five months. This is an enormous head start compared to outdated 13F information received 45 days later.
Detecting Portfolio Constituents
Another strategy that doesn’t follow the time constraints of regulatory followings has been produced by recent academic efforts that yielded an ability to estimate portfolio constituents within an 88-98% accuracy range using machine learning algorithms. This is called Fund Asset Inference and requires the aggregate value of a known portfolio in the form of a time series recording. This is helpful if firms know exactly which portfolio it intends to imitate but can lead to a narrow view of the market as it focuses on one portfolio manager at a time.
Detecting Order Types in Real-Time
Sentiment analysis and revealing portfolio constituents are helpful additions to existing strategies, but a broader and faster approach is the ability to detect hidden orders on the market placed by institutional investors, specifically iceberg order detection. Exegy-Signum’s Liquidity Lamp can show users when asset positions are moving in bulk, even when hidden order types attempt to minimize the impact of the trades on asset prices.
Since Liquidity Lamp operates in real-time on every stock on every US exchange, it provides instantaneous visibility into significant position changes. Aggregating the buying and selling activity using detected reserve orders across multiple venues can reveal the net movements of institutional positions, including short positions when selling overtakes buying. As a service, Exegy computes a daily aggregation of reserve order activity on a per stock, per exchange basis and delivers a summary file prior to the start of the next trading session, so no waiting around for regulatory filings to be published.
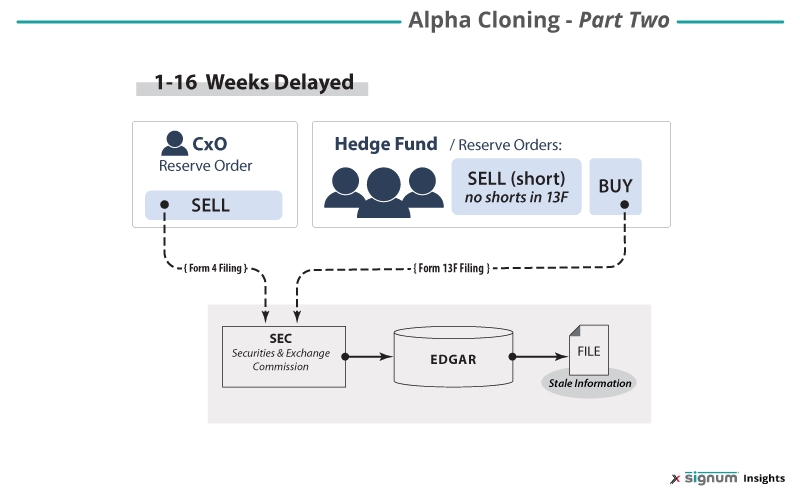
Getting Started
Instead of relying on complicated statistical analysis using delayed, alpha cloning strategy developers can implement one or more machine learning methods that provide up to date analysis. Firms with data scientists and the available resources can develop their own replicator models in-house or look to third-party providers.
Exegy’s Liquidity Lamp offers an economical option, saving time and allowing firms to repurpose personnel, while appealing to investors interested in the latest AI-driven methods. As asset managers adopt machine learning to enhance their portfolios it is only fitting that those following close behind them implement the same tech. For those interested in the future of artificial intelligence backed solutions, review our white papers, request demo data, or contact us for more ways we can serve your individual needs.
Want to know more? Learn how Liquidity Lamp Summary data can be used in alpha cloning strategies to create uncorrelated alpha.